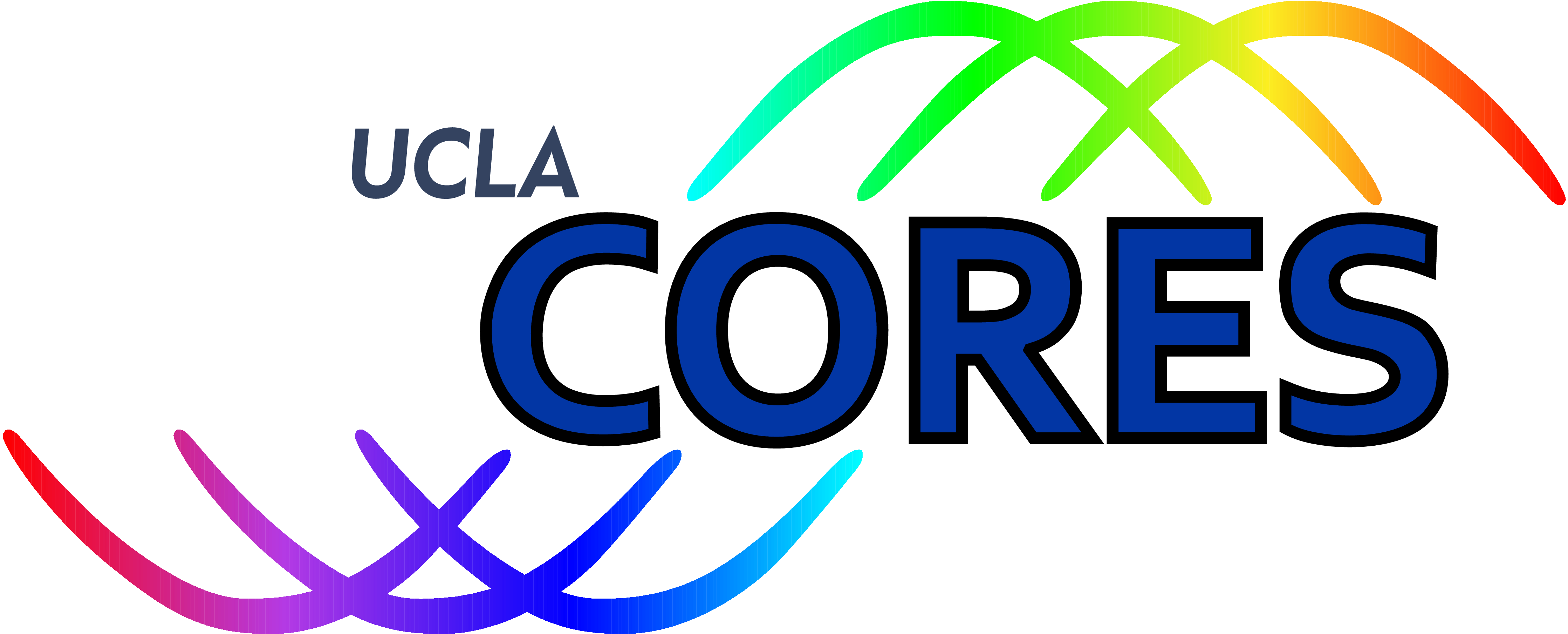
Cognitive Reconfigurable Embedded Systems Lab
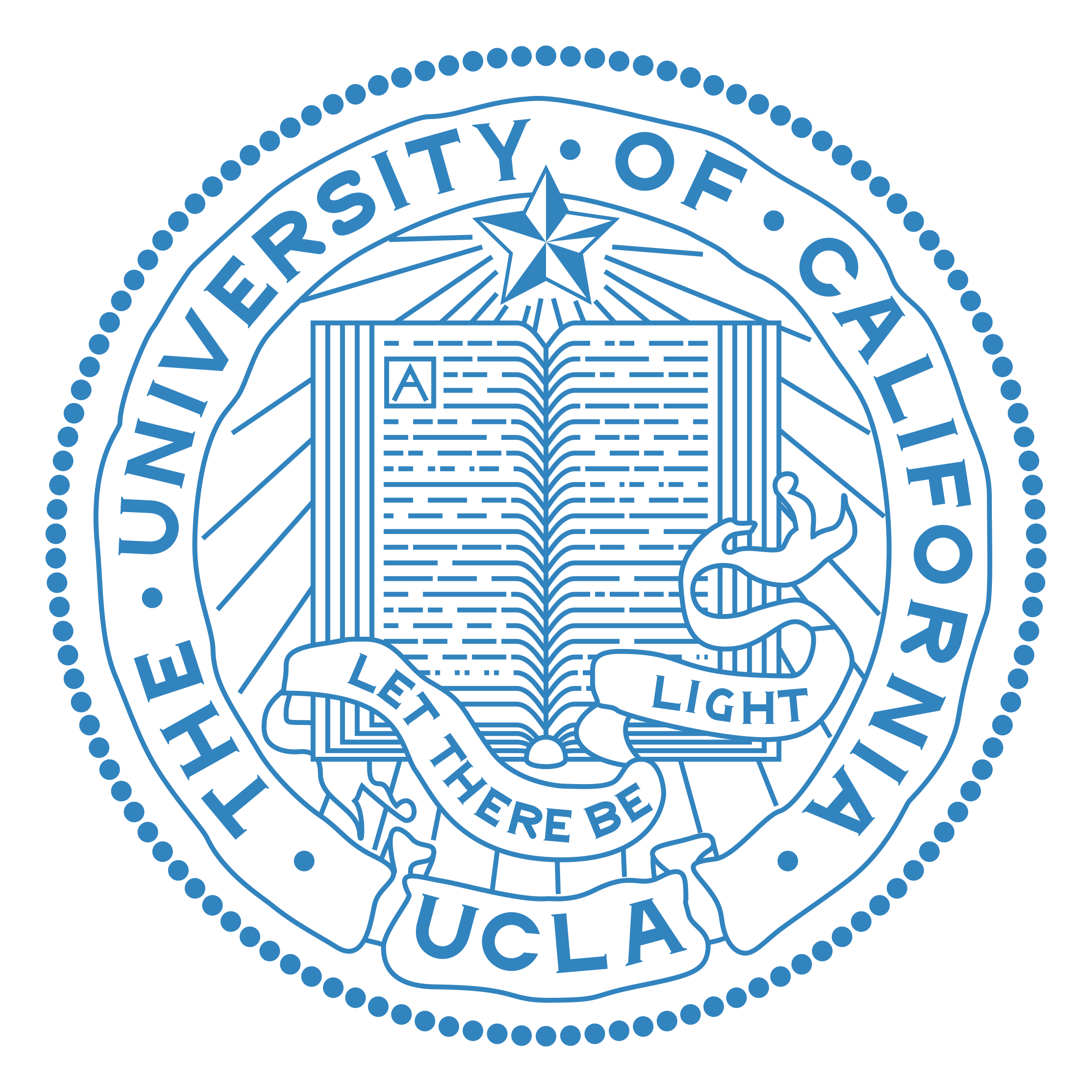
Machine learning for wireless networks co-existence and security
Another frontier in our research portfolio is aimed at developing machine learning framework to provide higher layer awareness among co-existing networks and enhance security. The widespread deployment of wireless devices poses new challenges in spectrum management and coexistence between heterogeneous devices. The first step in improving coexistence between different devices requires understanding the nature of signals occupying the spectrum. The large number of possible waveforms along with impairments added by the channel and the radio hardware make this problem challenging and drive the need for machine learning approaches to address it. We have developed a blind signal identification approach based on deep learning using only a short sequence of received samples that achieved the recovery of its symbols without any prior knowledge about the signal [8]. While spectrum sharing enables more flexible and efficient usage of spectrum, it also opens possibilities for new types of security attacks. We have considered the problem of transmitter authorization based on their physical layer fingerprints, and developed deep learning algorithm that exploits power amplifier nonlinearity fingerprint for robust recognition of rouge devices [9]. More recently, we are considering an open set scenario and anomaly detection, where it is further required to differentiate authorized transmitters from unauthorized ones.
Our research has been supported through
Projects
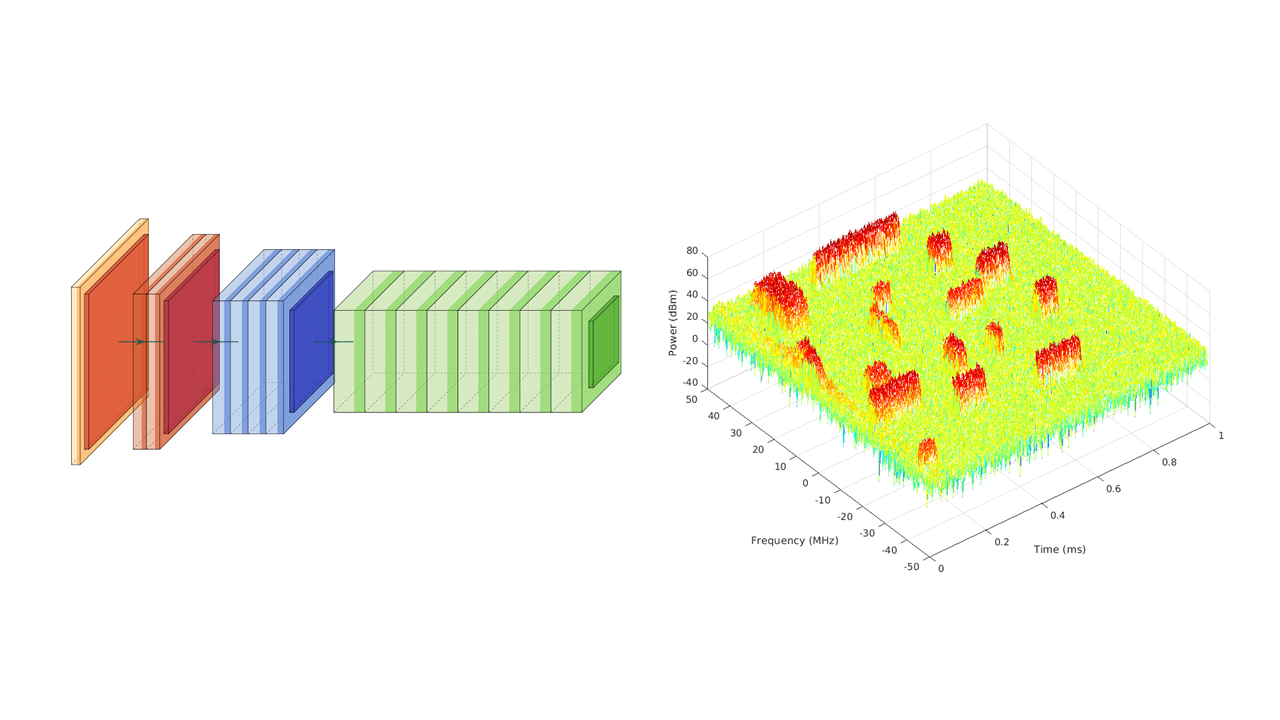