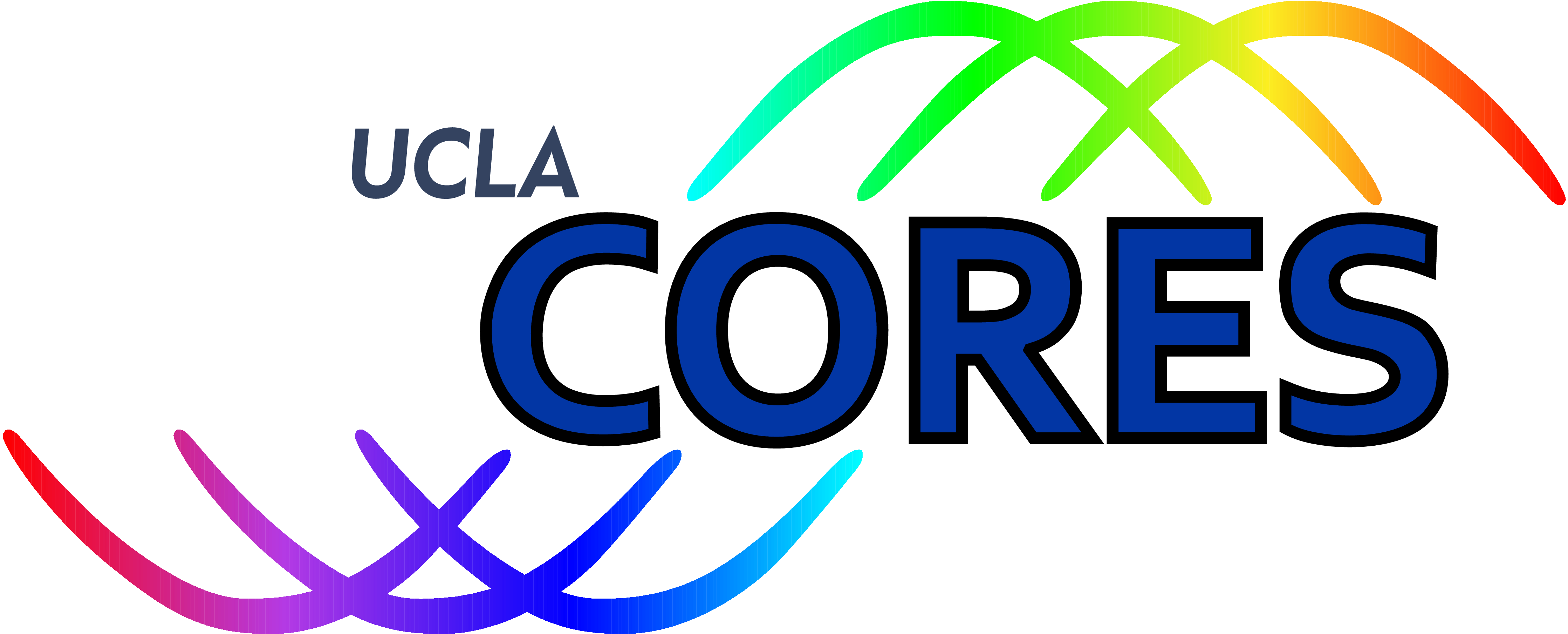
Cognitive Reconfigurable Embedded Systems Lab
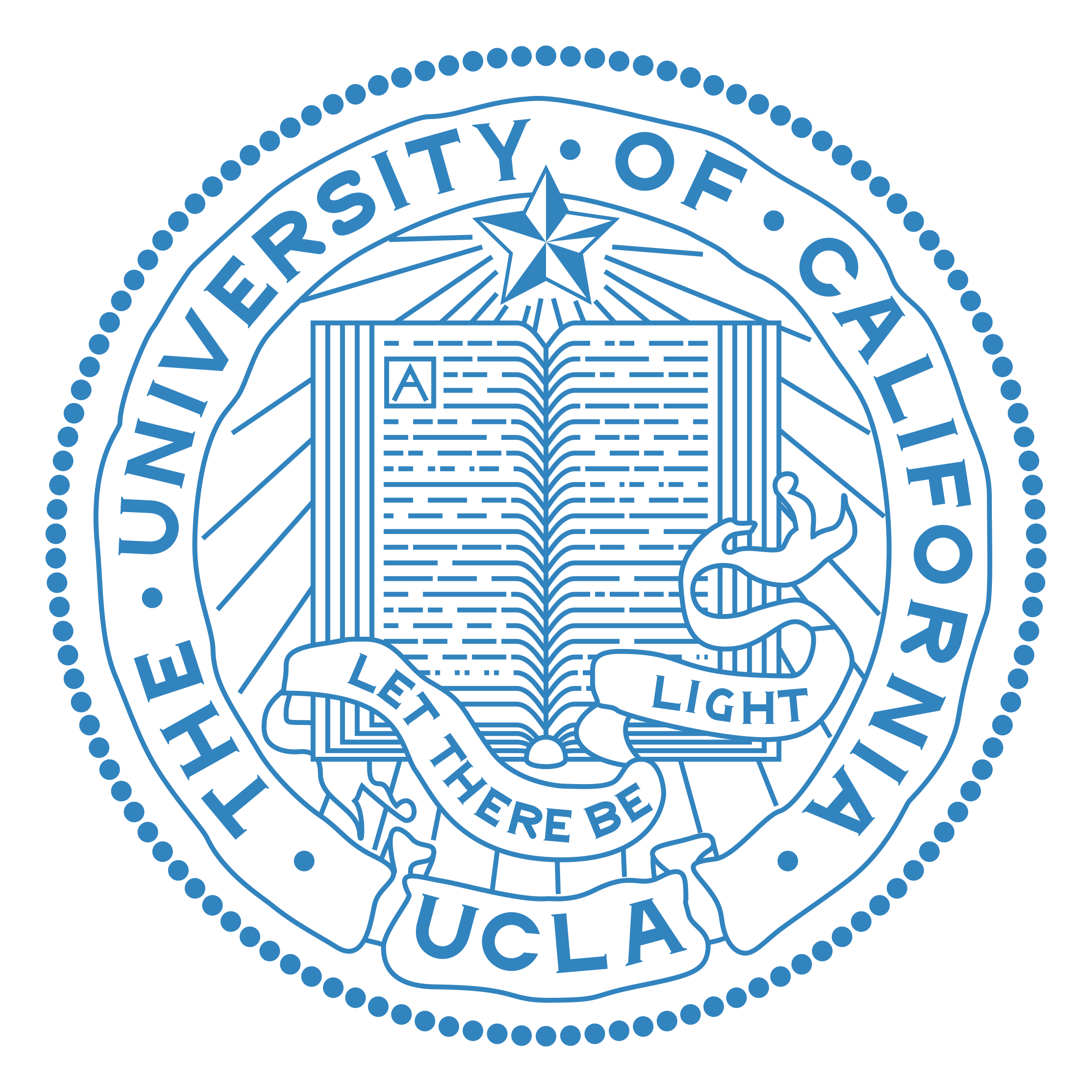
Machine Learning Assisted Beam Training and Tracking
In this project, we aim to reduce the beam alignment overhead for practical mmW communication through novel algorithms that combine compressed sensing and machine learning techniques. From compressed sensing, we propose pseudorandom beam designs to provide more angle of arrival information from fewer measurements. We then propose machine learning algorithms to solve for the complex relationship between pseudorandom beam measurements and the optimal pointing direction required for communication. Data driven methods are required for this problem, since realistic per-unit hardware variations create substantial deviations of the real antenna patterns from those predicted by theory. Using Facebook Terragraph 60 GHz channel sounders, we verify our mmW algorithms experimentally. Our initial work with static beam alignment is very promising, showing up to 90% overhead reduction with experiment data.
5G and future cellular technology will rely on millimeter-wave (mmW) communications to achieve extreme data rates. These high frequency mmW systems require narrow beams from large phased arrays and thus face the challenge of efficient beam alignment, or pointing the base station and mobile unit antenna patterns towards each other. Traditional designs use an exhaustive beam sweep, testing each possible pair of beams, to choose the best pointing direction. However, testing a large number of beam pairs would introduce a large communication overhead, increasing latency and decreasing effective data rates.
Staff
- Principal Investigator: Danijela Cabric
- Students: Han Yan, Benjamin Domae, Donar Li