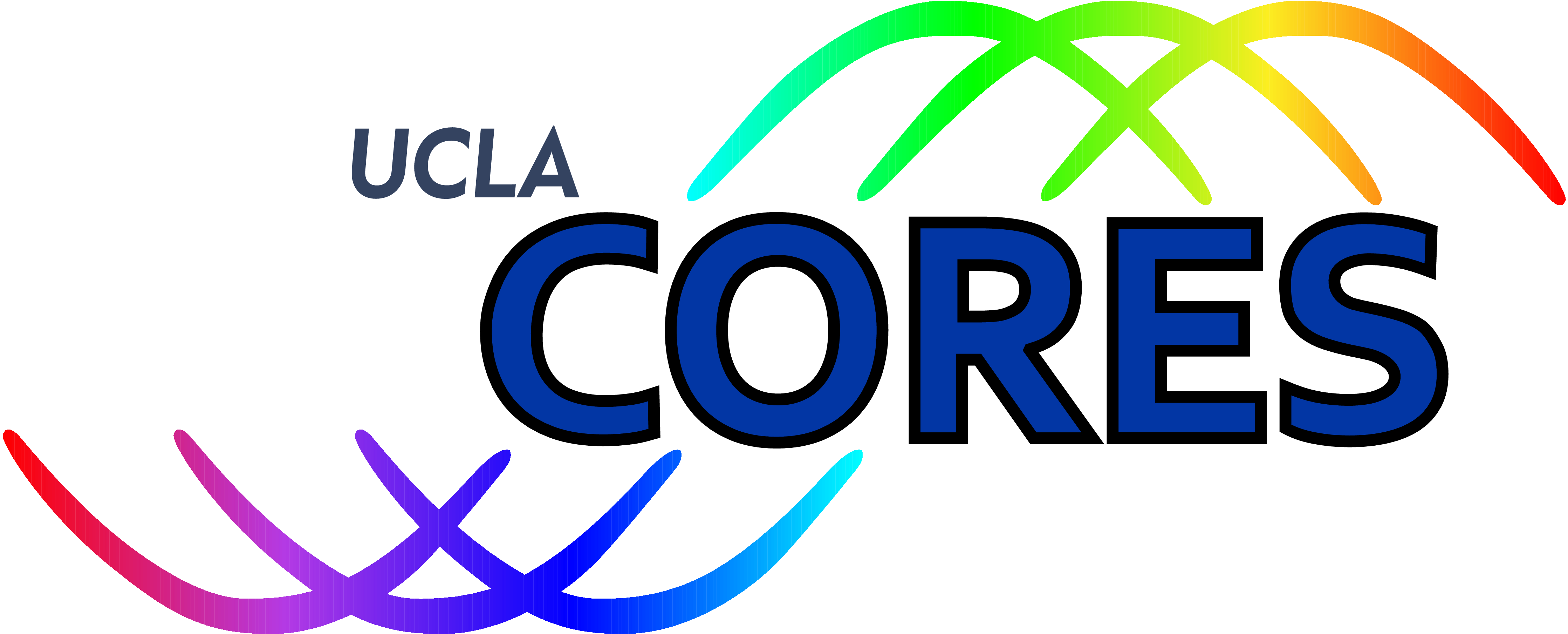
Cognitive Reconfigurable Embedded Systems Lab
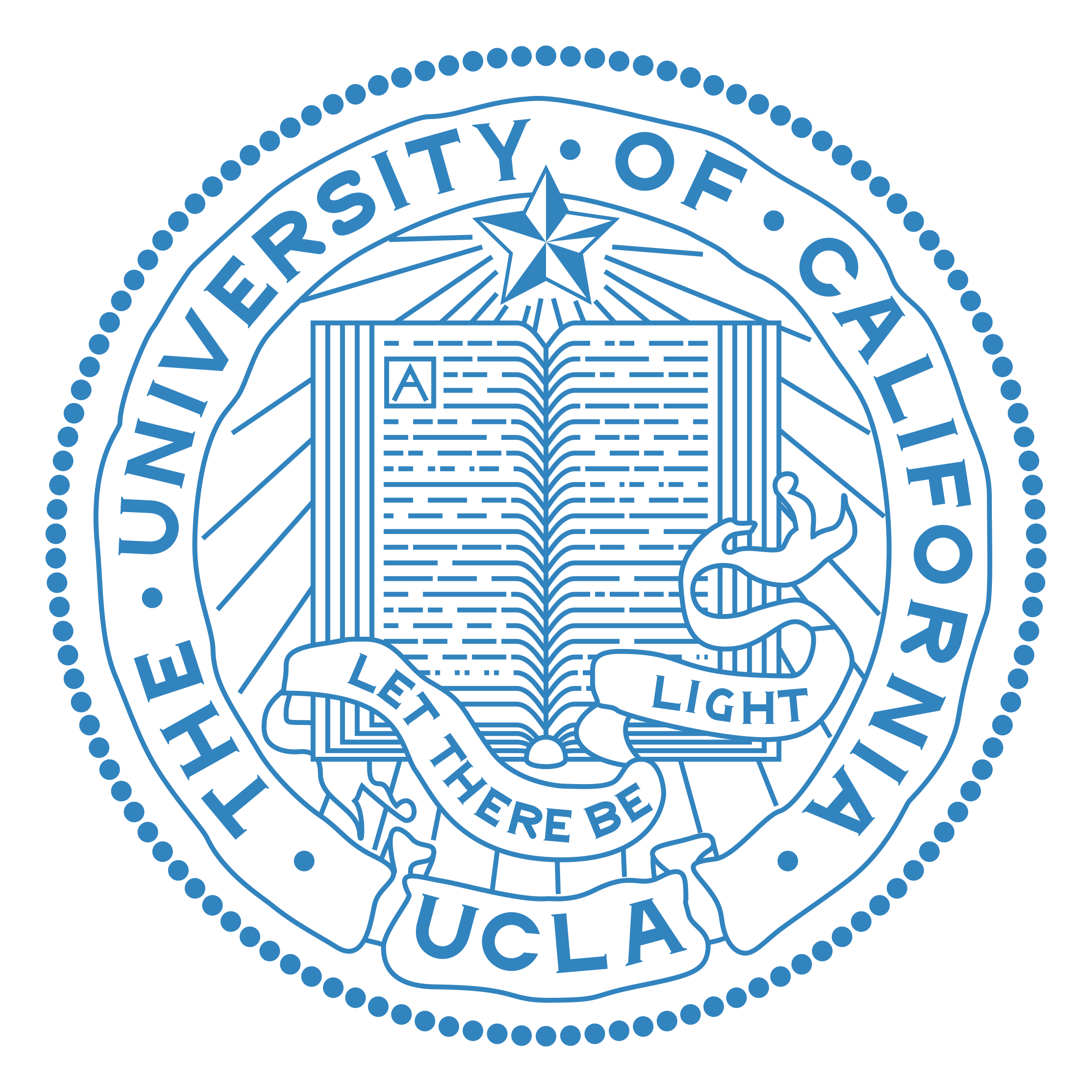
Machine Learning for Transmitter Authorization based on RF Fingerprints
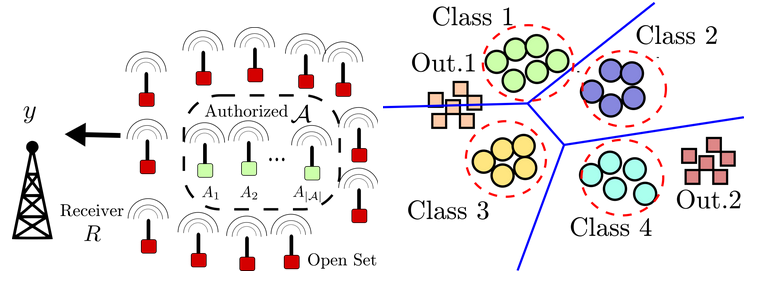
With the widespread adoption of the Internet of Things (IoT), the number of wirelessly connected devices will continue to proliferate over the next few years. Along with this increase, device authentication will become more challenging than ever specially for devices under stringent computation and power budgets. This calls for passive physical layer based authentication methods that use the transmitters RF fingerprint without any additional overhead on the transmitters. RF fingerprints arise from the transmitters’ hardware variability and the wireless channel and hence are unique to each device. Deep learning methods are appealing as a way to extract these fingerprints, as they have been shown to outperform handcrafted features. Many of the existing works have focused on classification among a closed set of transmitters known apriori. However, an intruder can be any device outside of this set. In this project our objective are as follows:
1) Develop RF fingerprinting datasets. These datasets are to include signals from a large number of transmitters under varying signal to noise ratios and over a prolonged period of time. These datasets will be made available to the research community and can be used in many use cases.
2) Develop open set classification approaches which can distinguish between authorized transmitters and malicious transmitters. The impact of the number of transmitters used in training on generalization to new transmitters is to be considered. Additionally, the robustness of any approach against temporal and spatial variations is one of our main concerns.
Staff
- Primary Investigators: Danijela Čabrić
- Students: Samer Hanna, and Samurdhi Karunaratne