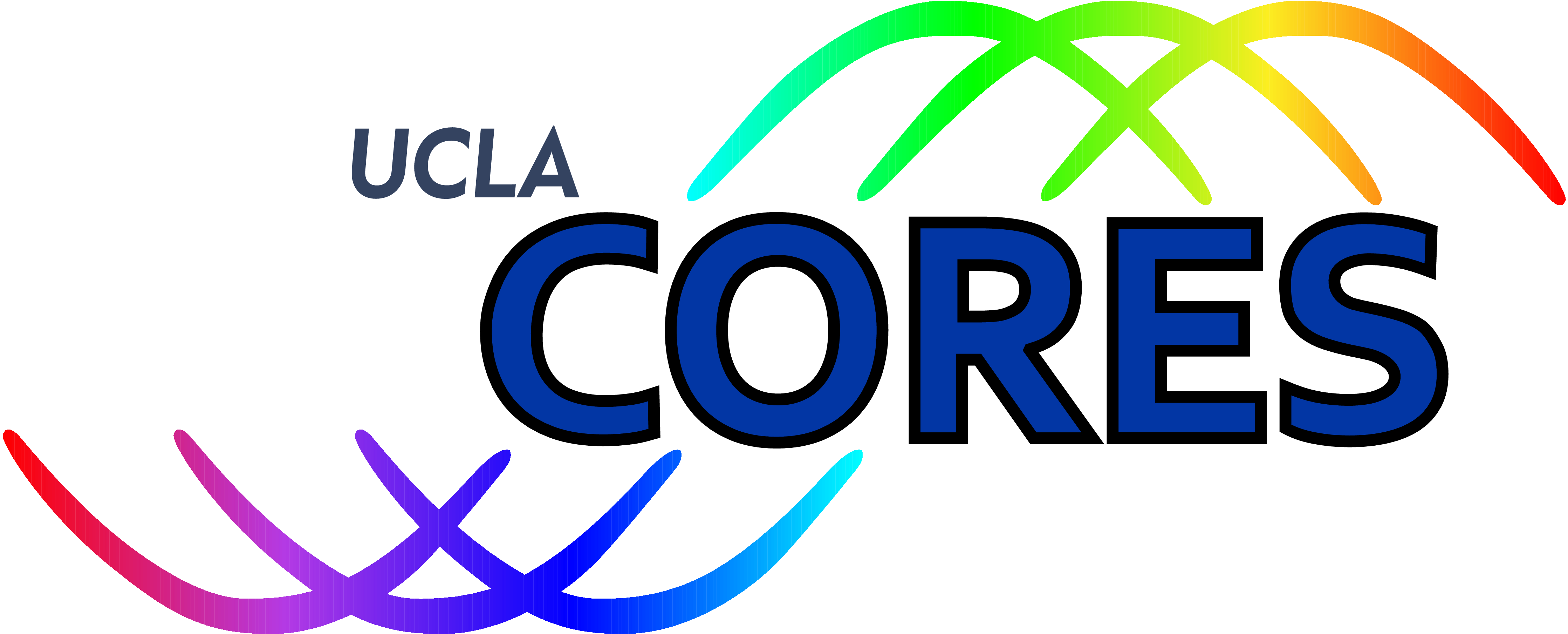
Cognitive Reconfigurable Embedded Systems Lab
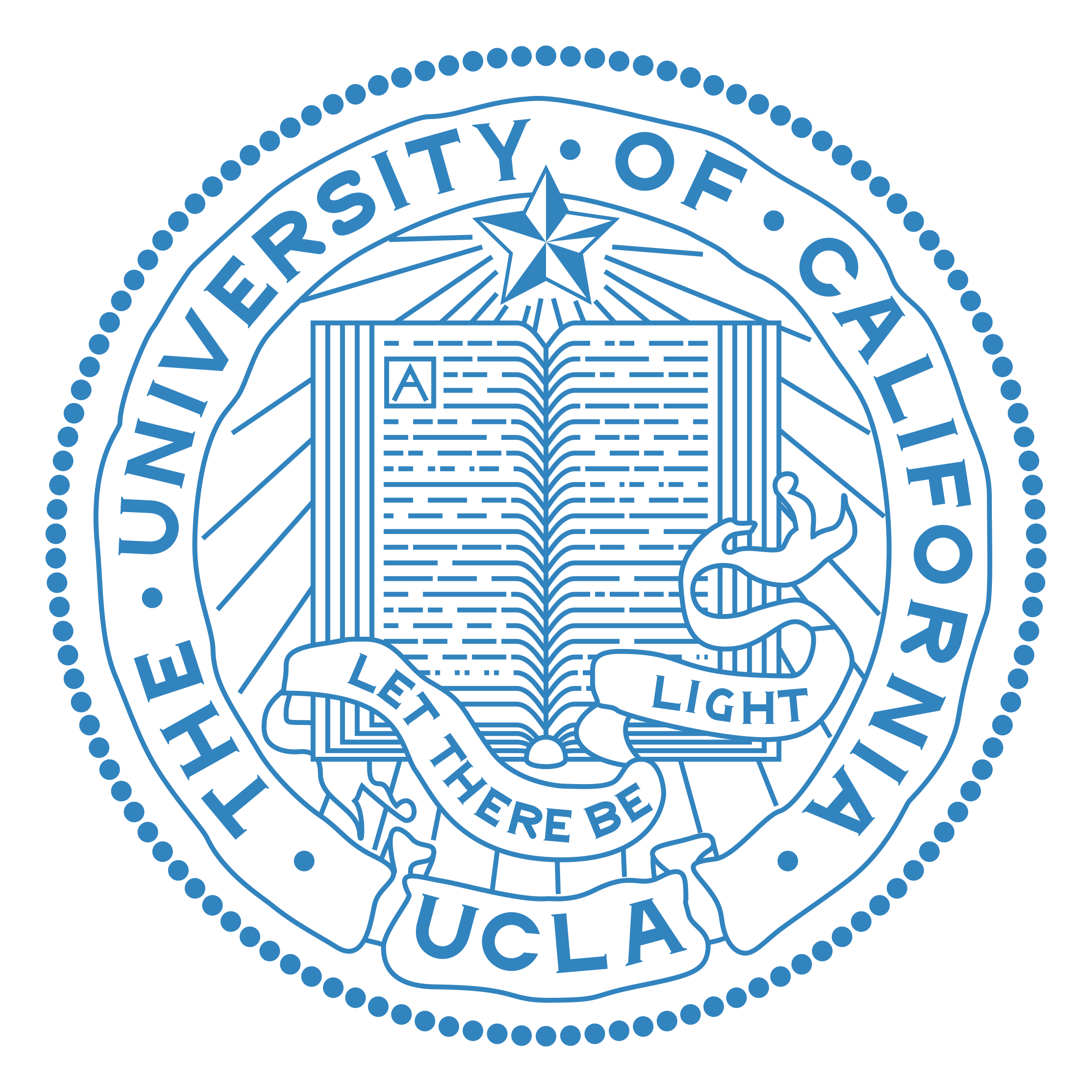
Deep Learning Aided Wideband Spectrum Sensing and Monitoring
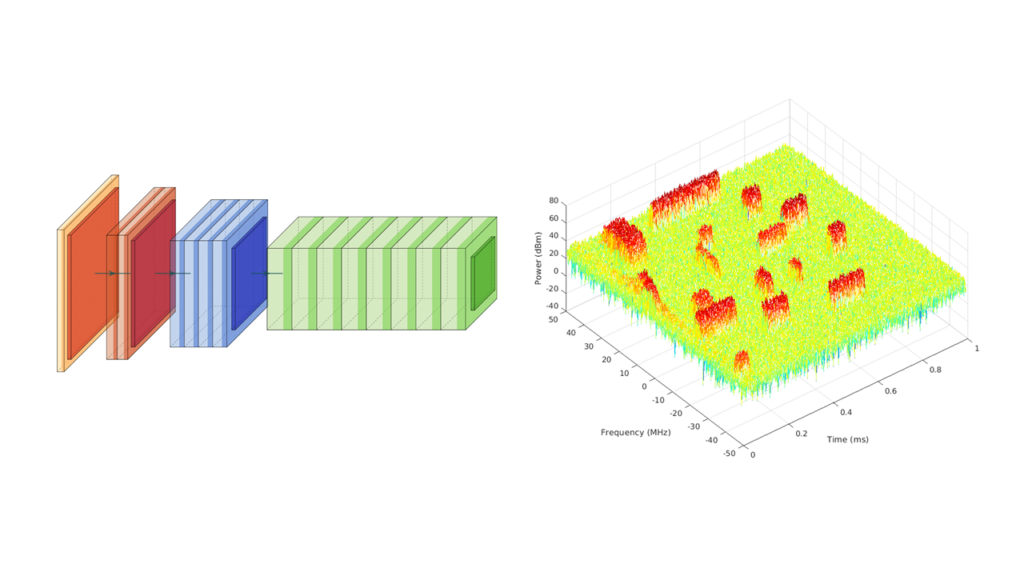
An electromagnetic spectrum can reveal the radio frequency (RF) threats in a given geographic location. Detecting and characterizing those RF footprints in the spectrum is essential to improve situational awareness and threat identification, prevention and response. Traditionally, digital signal processing techniques can be utilized for transmission detection, but those methods require prior knowledge about the signals such as wireless protocols. Moreover, any further analysis of the signals cannot be done in parallel with detection, which increases the latency of the approach. On the other hand, deep learning algorithms are able to extract complicated features from a signal, and yield multiple predictions simultaneously.
In this project, we want to address the following challenges:
- We develop RF signal datasets. The datasets are to mimic a realistic congested RF environment spanning a wideband, including signals of different properties, such as wireless protocols and signal to noise ratios.
- We develop deep learning algorithms to detect the RF signals without any prior knowledge. The algorithms need to accurately spot and localize the signals in time and frequency domains, for both known and unknown signal properties.
- On top of detection, we develop deep learning algorithms to identify the authority of the transmitters. The algorithms need to classify a known transmitter and learn the features of an unknown transmitter to track its appearance in the future.
- We integrate the developed algorithms into an end-to-end framework, which can be deployed on hardwares and operate in real time.
Sponsors
- SpectrumX
- STTR: AFRL/Perceptronics Solutions
Staff
- Primary Investigator: Danijela Čabrić
- Students: Tianyi Zhao and Benjamin Domae