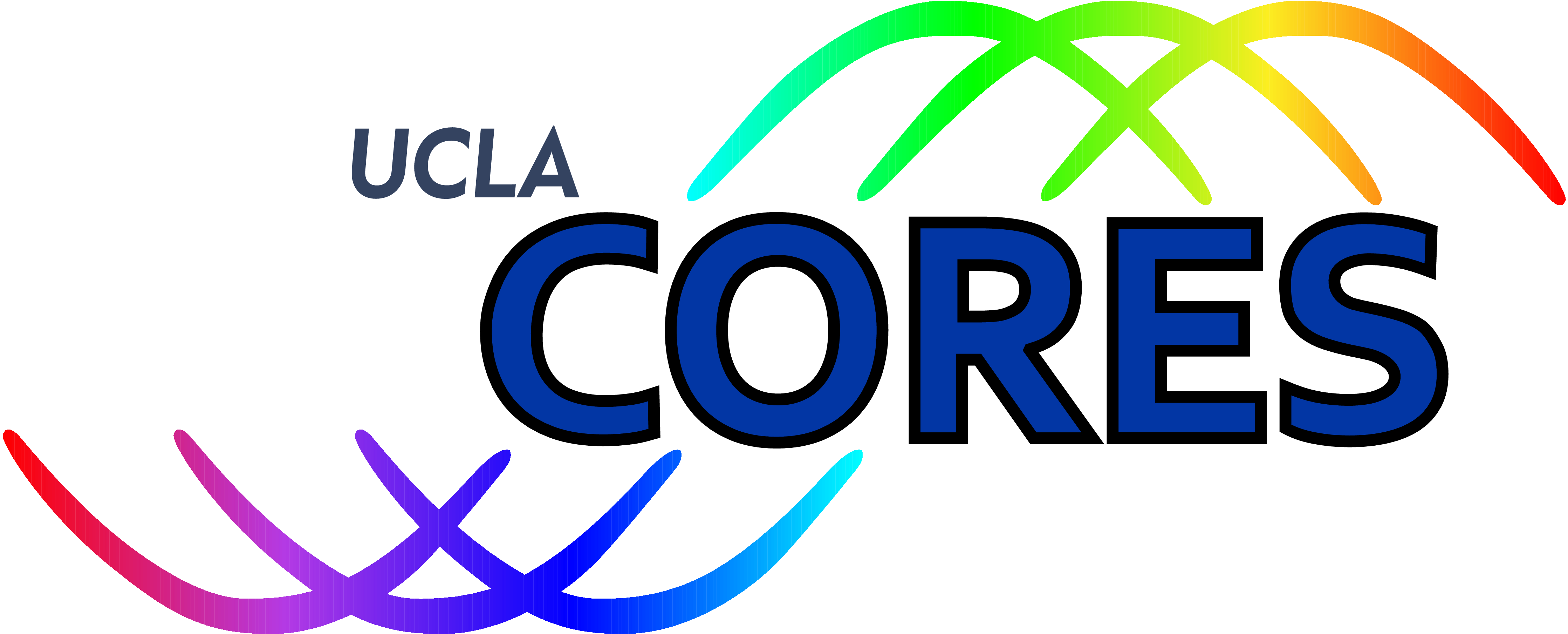
Cognitive Reconfigurable Embedded Systems Lab
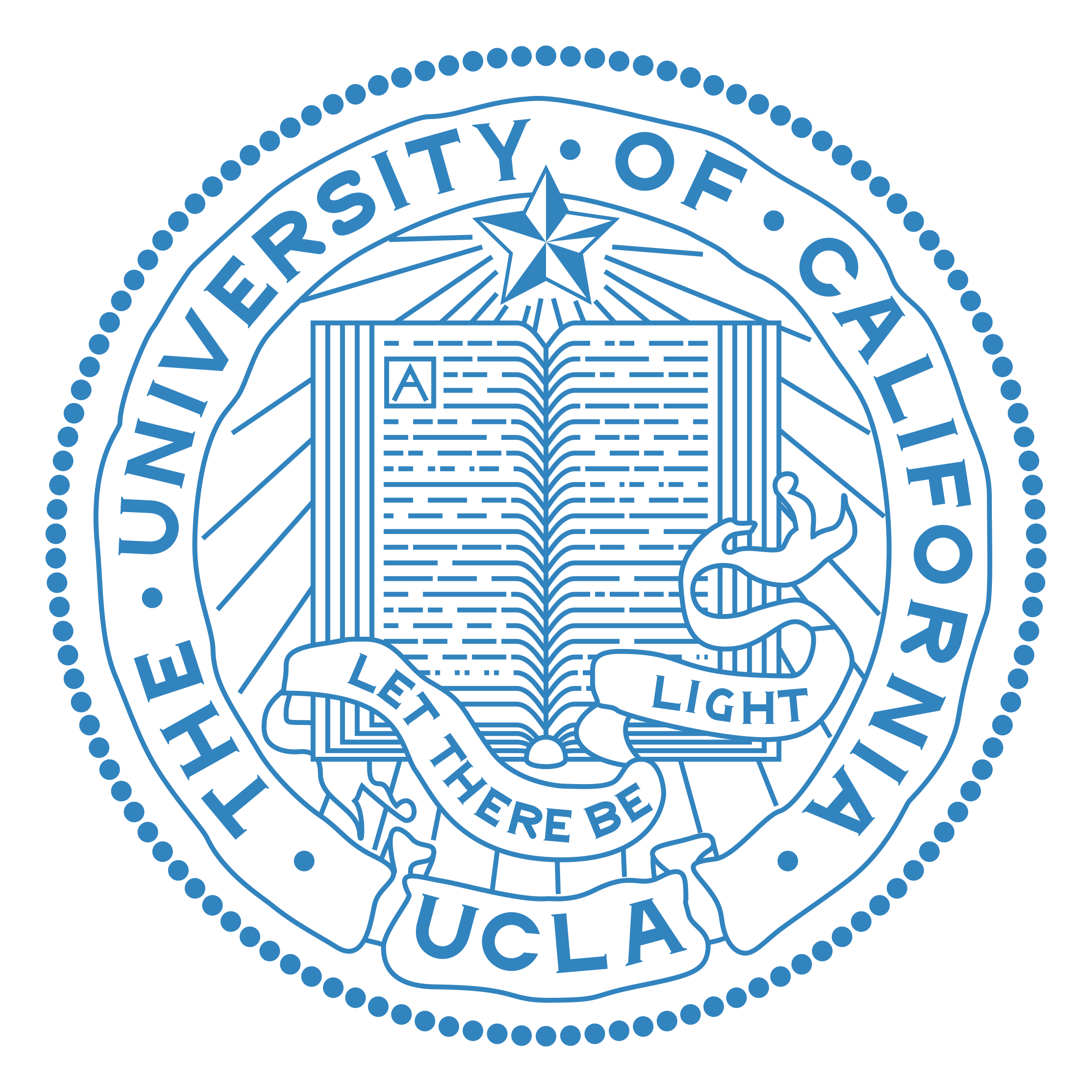
Machine Learning Based Physical Layer and Mobility Management Solutions Towards 6G
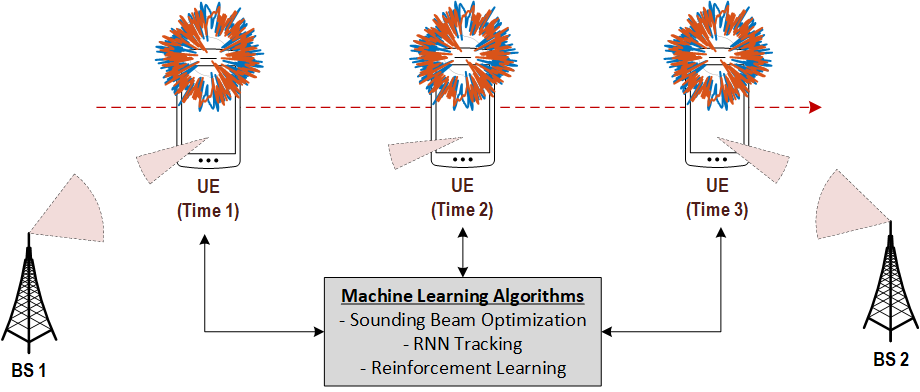
5G networks and 6G plans are embracing millimeter-wave (mmW) and sub-terrahertz (sub-THz) bands to unlock additional spectrum and to meet rising consumer demand for high-rate, mobile, cellular data. However, wireless systems at these bands face significant technical challenges to achieve their potential capacity and latency. Many of these challenges arise from the large antenna arrays required to overcome high path loss for mmW and sub-THz networks. Base stations (BSs) and user equipment (UEs) need to quickly point and track extremely narrow beams from their antenna arrays, even with high mobility, to maintain their links. In this project, we address the challenges of high communications overhead and latency, robustness to hardware impairments, and frequency handovers between base stations using machine learning (ML) techniques and real-world experiments on state-of-the-art testbeds. This work is split into three main thrusts:
- ML-assisted Beam Training, Beam Tracking, and Sounding Beam Optimization: This task uses neural network, recurrent neural network (RNN), reinforcement learning, and compressed sensing algorithms to reduce beam alignment and link maintenance overhead. We additionally design optimized beams for these applications.
- ML-assisted Physical Layer Receiver Correction: In this task, we explore neural network algorithms to remove distortion from realistic mmW and sub-THz receiver front-ends.
- ML-based Handover Management under Mobility: This task incorporates deep neural network and reinforcement learning algorithms to improve the efficiency of network handovers and reduce connectivity disruptions.
Sponsors
- NSF grant title: NSF-AoF: CNS Core: Small: Machine Learning Based Physical Layer and Mobility Management Solutions Towards 6G
- NSF Awards number: 2224322 [Link]
Staff
- Primary Investigator: Danijela Čabrić
- Students: Benjamin Domae, Donar Li, Enes Krijestorac, and Ibrahim Pehlivan
- Tampere University collaborators: Prof. Mikko Valkama, Jukka Talvitie, Roman Klus, Mehmet Ilter
Dissemination
- May 2023 Prof. Cabric included several lectures introducing students to mmW networks and array processing in the UCLA graduate course ECE 233 wireless communication system design, model, and implementation.
Publications
- B. W. Domae, V. Boljanovic, R. Li, D. Cabric, “Machine Learning Prediction for Phase-less Millimeter-Wave Beam Tracking,” in the 23rd IEEE International Workshop on Signal Processing Advances in Wireless Communications (SPAWC), July 2022
[Paper]
Data management
Research results and data from our works are available upon request.